Transforming Natural Language Processing
Admin / July 31, 2024
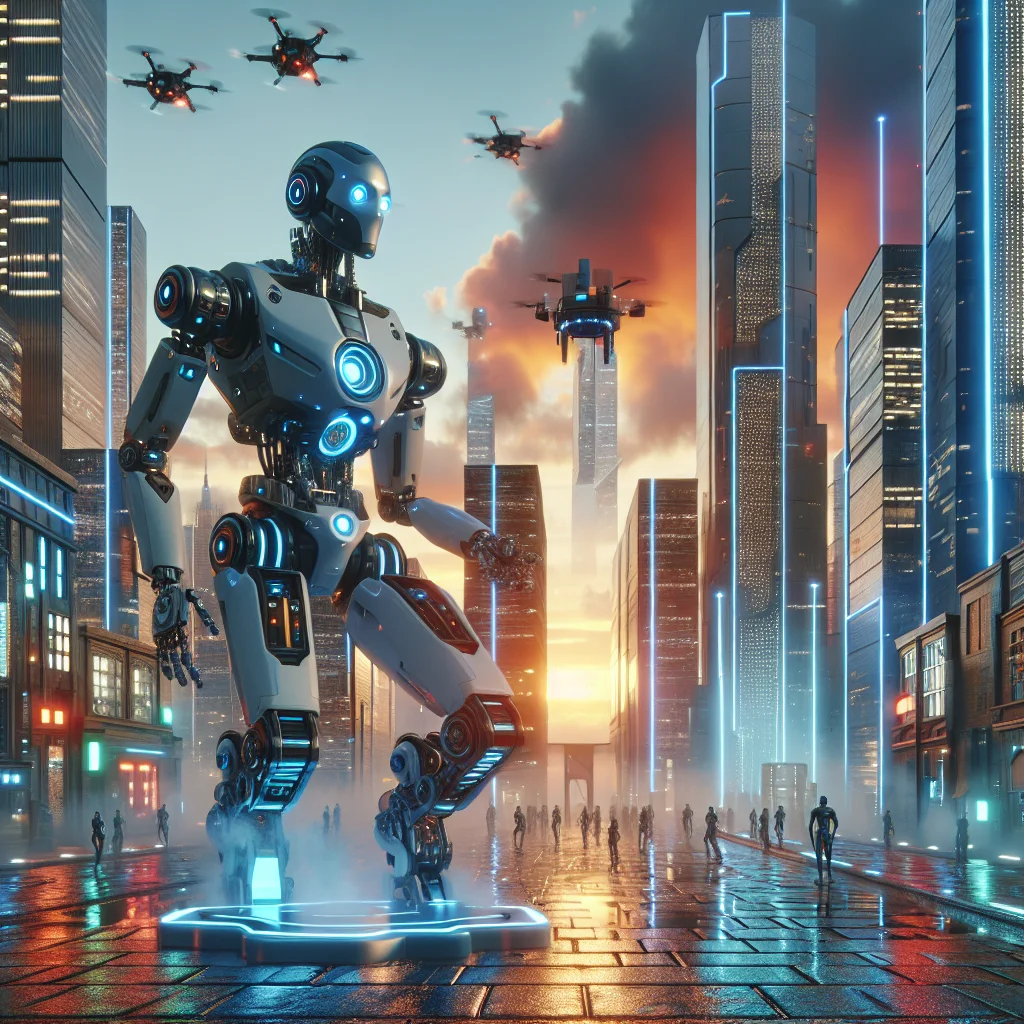
The landscape of natural language processing (NLP) has
undergone a remarkable transformation over the past few decades, primarily
driven by advancements in deep learning. As I delve into this subject, I cannot
help but reflect on how machine learning has significantly enhanced the
capabilities of natural language processing. This evolution is not merely an
incremental improvement; it represents a paradigm shift in how machines
understand and generate human language. The integration of machine learning techniques
has empowered NLP systems to process vast amounts of text data, enabling them
to learn patterns and nuances that were previously unattainable.
At the core of this transformation lie neural networks, which have emerged as the backbone of deep learning applications. These complex architectures mimic the human brain's interconnected neuron structure, allowing for sophisticated computations that facilitate language understanding. The ability of neural networks to learn from large datasets has driven advancements in various NLP tasks, such as sentiment analysis, machine translation, and text summarization. As I explore the evolution of language models, I am continually amazed by how these technologies have reshaped our interaction with machines, making communication more intuitive and efficient.
At the core of this transformation lie neural networks, which have emerged as the backbone of deep learning applications. These complex architectures mimic the human brain's interconnected neuron structure, allowing for sophisticated computations that facilitate language understanding. The ability of neural networks to learn from large datasets has driven advancements in various NLP tasks, such as sentiment analysis, machine translation, and text summarization. As I explore the evolution of language models, I am continually amazed by how these technologies have reshaped our interaction with machines, making communication more intuitive and efficient.
Understanding Neural Architectures
As I examine the intricacies of neural architectures, it
becomes evident that machine learning serves as a powerful enhancer for natural
language processing. The synergy between machine learning and neural networks
has led to the development of models that can comprehend context, semantics,
and even the subtleties of tone and style in language. This understanding is
crucial for applications that require a high degree of accuracy, such as
chatbots and virtual assistants, which rely on the ability to interpret user
intent effectively.
Deep learning has revolutionized the way autonomous systems operate in language understanding. By leveraging vast amounts of data and sophisticated algorithms, these systems can now engage in conversations, answer questions, and even generate human-like text. The implications of this are profound, as it allows for the creation of applications that can interact with users in a more natural manner, thereby enhancing user experience and satisfaction. As I reflect on these advancements, I recognize that the ongoing development of neural architectures will continue to push the boundaries of what is possible in the realm of natural language processing.
Deep learning has revolutionized the way autonomous systems operate in language understanding. By leveraging vast amounts of data and sophisticated algorithms, these systems can now engage in conversations, answer questions, and even generate human-like text. The implications of this are profound, as it allows for the creation of applications that can interact with users in a more natural manner, thereby enhancing user experience and satisfaction. As I reflect on these advancements, I recognize that the ongoing development of neural architectures will continue to push the boundaries of what is possible in the realm of natural language processing.
Challenges in Language Processing
Despite the remarkable progress made in natural language
processing, challenges remain that must be addressed to fully realize the
potential of machine learning in this field. One significant limitation is that
the accuracy of natural language processing systems can be adversely affected
by the constraints of machine learning algorithms. These algorithms may
struggle with ambiguity, idiomatic expressions, and cultural nuances, which can
lead to misunderstandings in language interpretation. As I consider these
limitations, it becomes clear that ongoing research and innovation are
essential to overcoming these hurdles.
Furthermore, neural networks require vast amounts of data for effective training. The quality and quantity of data play a crucial role in determining the performance of NLP models. In many cases, obtaining labeled data can be a daunting task, and the lack of sufficient training data can hinder the development of robust language processing systems. As I contemplate these challenges, I am reminded of the importance of continuous advancements in data collection and annotation techniques, which are vital for enhancing the accuracy and reliability of NLP applications.
Furthermore, neural networks require vast amounts of data for effective training. The quality and quantity of data play a crucial role in determining the performance of NLP models. In many cases, obtaining labeled data can be a daunting task, and the lack of sufficient training data can hinder the development of robust language processing systems. As I contemplate these challenges, I am reminded of the importance of continuous advancements in data collection and annotation techniques, which are vital for enhancing the accuracy and reliability of NLP applications.
Applications of Deep Learning in NLP
The applications of deep learning in natural language
processing are vast and varied, showcasing the profound impact of this
technology on the field. Deep learning enhances machine learning algorithms,
allowing for more sophisticated analysis of text data. For instance, in
sentiment analysis, deep learning models can discern the emotional tone of a
piece of text with remarkable accuracy, providing valuable insights for
businesses and organizations. This capability is instrumental in understanding
customer feedback, social media sentiment, and market trends.
Moreover, neural networks have enabled the development of autonomous systems in language understanding, such as virtual assistants and chatbots. These systems can process natural language queries and respond in a way that feels conversational and engaging. The ability of deep learning models to generate contextually relevant responses has transformed customer service, making interactions more efficient and effective. As I explore these applications, I am continually impressed by how deep learning has not only enhanced the functionality of NLP systems but also revolutionized the way we communicate with technology.
Moreover, neural networks have enabled the development of autonomous systems in language understanding, such as virtual assistants and chatbots. These systems can process natural language queries and respond in a way that feels conversational and engaging. The ability of deep learning models to generate contextually relevant responses has transformed customer service, making interactions more efficient and effective. As I explore these applications, I am continually impressed by how deep learning has not only enhanced the functionality of NLP systems but also revolutionized the way we communicate with technology.
Future Trends in Autonomous Language Systems
Looking to the future, I am excited about the advancements
in machine learning that will further enhance the capabilities of autonomous
systems in natural language processing. As researchers continue to innovate and
refine algorithms, we can expect to see significant improvements in the
accuracy and efficiency of language models. These advancements will likely lead
to more sophisticated applications that can understand and generate human
language with even greater precision.
Deep learning is poised to revolutionize natural language processing applications globally. The potential for applications in various industries, from healthcare to finance, is immense. As I consider the future landscape of NLP, I envision a world where machines can seamlessly integrate into our daily lives, understanding and responding to our needs in real-time. This vision is not merely aspirational; it is grounded in the ongoing advancements in deep learning and machine learning that continue to push the boundaries of what is possible in natural language processing. The journey of transforming language processing through deep learning is one that I eagerly anticipate, as it promises to redefine our interactions with technology in profound ways.
Deep learning is poised to revolutionize natural language processing applications globally. The potential for applications in various industries, from healthcare to finance, is immense. As I consider the future landscape of NLP, I envision a world where machines can seamlessly integrate into our daily lives, understanding and responding to our needs in real-time. This vision is not merely aspirational; it is grounded in the ongoing advancements in deep learning and machine learning that continue to push the boundaries of what is possible in natural language processing. The journey of transforming language processing through deep learning is one that I eagerly anticipate, as it promises to redefine our interactions with technology in profound ways.